New Funding Approaches Call for a New Way of Measuring Impact
The focus of financial sector development is shifting. Development organizations funding financial inclusion now operate with a vision of sustainability, resilience and impact at scale, and their goals stretch beyond building individual institutions. Now, they aim to improve the whole ecosystem for financial services, taking a facilitative rather than a direct intervention approach.
The reason for this shift is the recognition that financial service markets are complex and dynamic. These dynamics are not fully predictable; it is not possible in advance, for example, to know how poor clients will react to a particular new product or how competitors adapt to a particular innovation introduced by a program. Similarly, dynamics are changing over time. A strategy that was developed at the beginning of a program might not be appropriate two years into implementation. Consequently, we cannot design an optimal solution up front.
Despite this shift and the appearance of some development funded programs that follow this rationale, there are many challenges on the road to financial inclusion. One of them is that programs struggle – or don’t even attempt – to measure their impact on a systemic level. Monitoring frameworks often haven’t caught up with the shift from direct intervention to facilitation approaches.
Secondly, current monitoring practices often emphasize measuring numbers of and effects on direct beneficiaries. While this surely is important information, we also need to recognize that the changes seen on a beneficiary level are due to changes in the structure and dynamics of the financial ecosystem. A high number of beneficiaries alone does not necessarily indicate that systemic change has happened. We need to find other ways to measure that.
Finally, the results agenda has put a strong pressure on donors to prove that the money they spend is used efficiently. This pressure is forwarded to implementing organizations. As a consequence, monitoring frameworks are still predominantly designed for accountability towards funders rather than as a management and learning tool for the programs themselves. Below are a few guiding principles for how to change this.
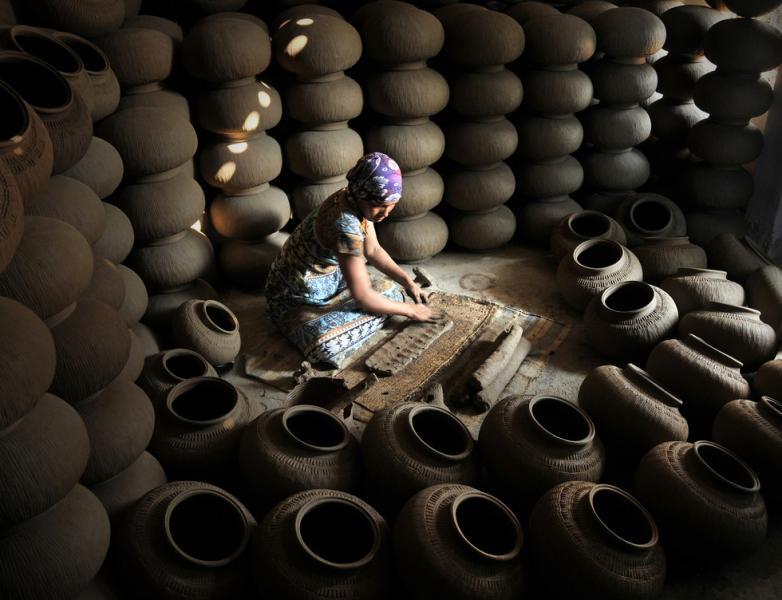
Photo Credit: Ady Agustian, 2014 CGAP Photo Contest
From proving theory to generating knowledge
Implicitly or explicitly, funders’ investments have a hypothesis on how to improve the financial market system. Good practice in monitoring is to make this hypothesis explicit in the form of a Theory of Change. This is good. But in many cases, the process of making hypotheses explicit leads to intricate results chains packed into enormous flow diagrams with proliferating boxes and causal connections. Indicators are developed for each box; the diagram becomes a blueprint and the indicators the basis for our monitoring efforts. Monitoring becomes an exercise in filling the cells of a complicated spreadsheet.
Reality is not so mechanical. Instead of gearing the monitoring system towards proving that our theory is correct, we should use it to generate more and better knowledge on what works and doesn’t work. There are often competing hypotheses on what works and no clear evidence to support one hypothesis over another. Instead of forcing consensus around one Theory of Change, we should be trying different things and seeing what effects they have.
From top-down to emergent
If a monitoring framework is focused on proving the validity of an initial Theory of Change, how this theory looks tends to dictate where program money is spent. But because optimal solutions cannot be designed based on analysis only, initial Theories of Change are influenced by what we believe, by our biases and ideologies.I suggest that the initial Theory of Change should be seen as a set of hypotheses that need to be tested rather than as a blue print for program implementation and monitoring.
Rigid monitoring systems incentivize program managers to stick to the original ‘plan’, spending more money to make it work. Testing hypotheses, learning, and adapting allows us to let solutions that work emerge from the context. Monitoring systems need to make the shift from measuring indicators that prove a theory to becoming a practical tool for program management to explore, learn and support emergent change.
From static to dynamic and adaptive
Monitoring frameworks often assume that program interventions lead to predictable results – outputs, outcomes, impacts – for which indicators can be defined. An initial baseline study is conducted, and over time indicators track changes against this baseline.
However, the reality is that financial inclusion programs don’t exist in a vacuum. Priorities shift, interventions change, and strategies may lead to unintended effects. This is why monitoring frameworks need adaptability and flexibility so that they can be responsive to and always generate relevant data when it is needed.
From spotlight monitoring to wider scanning for change
Because of the nature of financial markets, program interventions can lead to multiple effects – intended or unintended, positive or negative. Many factors beyond the specific program being measured are influencing financial inclusion at any given time. A program can only influence some of these factors. If we focus our monitoring efforts only on a set of indicators derived from a Theory of Change, which predicted specific pathways of change, we are blind to all the other effects of our interventions that we could not have predicted. We also don’t capture all the other factors that are also influencing our programme’s results. Hence, we need to complement indicator-based monitoring systems with broader observations that can capture wider system changes.
Comments
Can this programs reach
Can this programs reach Uganda?
Add new comment