Algorithm Bias in Credit Scoring: What’s Inside the Black Box?
As digital financial services (DFS) expand around the world with the promise of improving financially excluded customers' access to more affordable products and services, the growing use of algorithms opens opportunities but also the possibility for unfair bias and discrimination.
Using artificial intelligence, regression analysis and machine learning, developers and financial services providers are tapping into predictive algorithms that can make better and faster decisions than humans. Although well-developed algorithms can make more accurate predictions than people because of their ability to analyze multiple variables and the relationships between them, poorly developed algorithms or those based on insufficient or incomplete data can easily make decisions worse. Providers generally consider their algorithms to be confidential because they provide a competitive advantage, which makes it difficult to assess how they are making their decisions.

Regardless of the methodology used to develop them, algorithms are basically a set of rules built to solve a problem using a series of mathematical calculations. In financial services, algorithms are most commonly used to predict credit risk and accept or reject prospective borrowers. These algorithms can make decisions in seconds without any human interaction, so they are particularly relevant for issuing small-ticket loans for which low operation costs are critical to profitability. These algorithms estimate the probability that an applicant will default by comparing his or her current and historical data to data on borrowers who have taken similar loans in the past. An applicant is considered risky if people who share his or her characteristics and behaviors have paid late or defaulted.
Credit scoring algorithms are not new, but there are three reasons that it is becoming more important to take a critical look at them:
- The range of characteristics and behaviors that financial services providers can use in their algorithms is growing, thanks to the increased availability of consumer data.
- Credit scoring models are becoming more complex and automated, and there is less human oversight of the roles that various characteristics are playing in applicants’ final scores.
- Algorithms are becoming more common in emerging markets, where there is often less regulation and oversight of what data are used and how they are used.
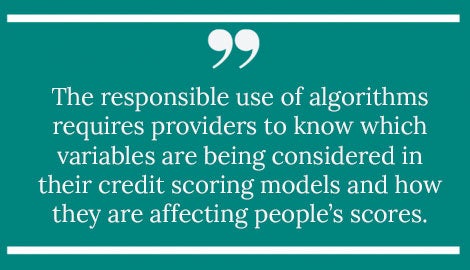
Algorithms and automation don’t necessarily imply a bigger risk of discrimination than traditional types of credit scoring. In the absence of algorithms and data-driven models, decisions on creditworthiness are made by loan officers. Consciously or unconsciously, people tend to have biased views based on the limited information at their disposal, and it’s hard for a company to ensure all its employees make unbiased assessments of applicants. One of the advantages of algorithms is that they can be developed, reviewed and monitored to avoid certain forms of discrimination. For example, you can tell your employees not to consider an applicant’s gender when making a loan decision, but can you be sure that they aren’t exhibiting a gender bias even at an unconscious level? With an algorithm, you can simply ensure a gender variable, and closely correlated variables are not included when computing a score.
The problem is that algorithms require people to ensure their impartiality. In developed markets, where credit bureaus exist and are widely used for scoring purposes, there are norms and regulations in place that prohibit the inclusion of specific data in algorithms. The main purpose of these regulations is to avoid the risk of algorithms being unfairly biased toward certain groups of people. In the United States, for example, data on an applicant’s race, ethnicity and closely correlated factors like ZIP code cannot be used to make a credit decision.
Other markets lack such safeguards. In the absence of regulation, it is difficult for financial services providers to agree on a universally accepted answer to what types of data should be included in credit scoring algorithms. Models can be developed in-house by financial service providers or outsourced to a developer under different types of arrangements. Either way, the provider is incentivized to maximize the predictive power of its model, regardless of what type of information the model uses to make predictions. This is especially true in cases when algorithms are outsourced and presented to DFS providers (and ultimately their customers) as black boxes. It also holds true in countries that don’t require providers to disclose what types of information were used to determine an applicant’s score.
. Algorithms will reflect the data that were used to build them. For example, immigrants may have low repayments rates due to their limited access to local networks, restrictions on their business activities and other factors. If this is the case and providers are allowed to use data that identifies applicants as immigrants, the algorithm may reinforce these applicants’ financial exclusion. Variables pointing to stability, like how long an applicant has been with his or her current employer or living at his or her current residence, provide another example. Stability generally helps a customer get a better score. However, this factor may put forcibly displaced people at a disadvantage. A provider could easily correct for this by not considering time at current residence for this segment, but this would require a developer to check and adjust for the bias.
In the context of developing financial markets, where regulations are limited and norms are not established, self-regulation would be a step in the right direction. Investors and supervisors can provide incentives for self-regulation by asking for information on the variables providers are using in their algorithms and how excluded groups are being treated. Even in absence of clear rules, financial services providers might think twice if they have to report to their supervisor or a potential impact investor that they reject 30 percent of male applicants and 60 percent of female ones. Can different stakeholders in the financial inclusion space work together to identify algorithms bias and introduce fixes when certain groups are being treated unfairly? Should standards be put in place to require greater transparency among algorithm developers and to create mechanisms for understanding and monitoring biases? Let us know what you think in the comments below.
Add new comment